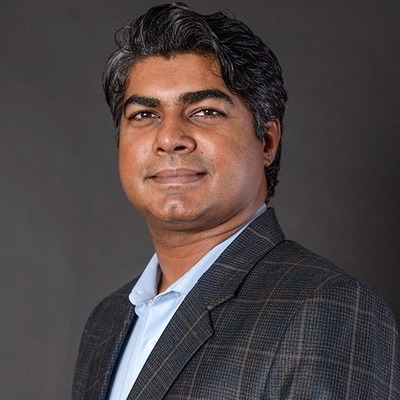
INSTRUCTOR: Siddharth Misra, PhD
DISCIPLINE: Geoscience, Engineering, Multi-Disciplinary & Introductory, Unconventional Reservoirs
COURSE LENGTH: 2 Days
CEUS: 1.6
AVAILABILITY: Public, In-House, & Live Online
COURSE DESCRIPTION: This course will provide working knowledge on using python programming and open-source packages essential for data analytics and machine learning. The entire course is based on live demos of codes and workflows in the Jupyter Notebook environment. The course will help geoscientists, geophysicists, and petroleum engineers learn python programming at a beginner to intermediate level. The course uses various types of data: well logs, core data, well performance data, and production data.
The focus of this course is on introducing Python programing skills that are pre-requisites to real-world data analysis. The course will not explore applications on large-sized field data.
LEARNING OUTCOMES:
- Assemble open-source coding and scripting workflows in Python to solve basic data science problems related to subsurface data.
- Apply numpy, pandas, matplotlib, seaborn and sklearn packages on subsurface data.
- Solve supervised regression problems using ElasticNet, random forest, nearest neighbor, and LASSO regressors.
- Solve supervised classification problems using nearest neighbor, random forest, and support vector classifiers.
- Solve unsupervised clustering problems using k-means and mean shift techniques.
- Apply anomaly detection and data preprocessing.
- Apply neural network and boosting methods.
- OPTIONAL: Learn about time-series forecasting, clustering, and spatial data analytics through two-week project.
OPTIONAL PROJECT FOR PRIVATE COURSE: At additional cost, a group project lasting for two weeks at the end of the course will help the participants try out the learned concepts by modifying the shared Jupyter Notebooks. The practice session will allow deeper interaction with the instructor on problems specific to the participants.
PROJECT OPTIONS FOR PRIVATE COURSE:
Client can select 2 of the following 3 projects according to the needs of the participants. Instructor will hold three 2-hour virtual sessions guiding the participants through the tasks over a 2-week period. The solutions will be reviewed in the final session.
- Production Forecasting
- Shale Image Analysis
- Clustering the Cross-Well Seismic Traces
Project Option #1: Production Forecasting (Part of Optional Two-Week Project)
Data contains the following 13 columns for 3 wells:
-
-
- DATE
- WELL_ID
- ON_HRS
- DOWN_PRES
- DOWN_TEMP
- PROD_TUBING
- CHOKE_SIZE
- WHPres
- WHTemp
- PROD_CHOKE_SIZE
- OIL_VOL
- GAS_VOL
- WAT_VOL
-
Task Description:
Forecast “Oil/Gas/Water Rates” T days in future as a function of the desired choke size and flow period in next T days given a historical trend of oil/water/gas rates, downhole pressure, downhole temperature, wellhead pressure, flow period and choke size for N days in the past.
-
-
- Controllable operational features: flow period and choke size
- Response features: Rates, pressures, and temperatures
- For example, when T = 1 day, there will be 10 features and 3 targets
-
Key Takeaways:
-
-
- Time-Series cross validation
- AdaBoost vs. Gradient Boosting
- Hyper-parameter tuning of neural network
- Model export and model deployment
- Pipelines
-
Project Option #2: Shale Image Analysis (Part of Optional Two-Week Project)
High-resolution microscopy image of shale samples
Task Description:
-
-
- Train classifier to locate matrix, pores, kerogen, and pyrite in multiple images
- Perform image compression using clustering
-
Key Takeaways:
-
-
- Image analytics packages: cv2 and skimage
- Filters and feature extraction
- Image compression
- Feature importance
- Classification model evaluation
-
Project Option #3: Clustering the Cross-well Seismic Traces (Part of Optional Two-Week Project)
Cross-well seismic imaging of a CO2 storage reservoir
Task Description:
-
-
- Perform feature extraction by applying Sobel, Hessian, Difference of Gaussian, Local Binary Pattern, and Wavelet Transform
- Use clustering to identify regions of distinct CO2 distribution and content
-
Key Takeaways:
-
-
- PIL and skimage packages
- Filters and feature extraction
- Optimum cluster numbers
- Silhouette method
- Davies-Bouldin index and Calinski & Harabasz criterion
-
COURSE CONTENT:
-
- 1 Hour: Using numpy on large arrays, using pandas on large tabular data
- 1.5 Hours: Using numpy and pandas on well data for:
- Data preprocessing
- Exploratory data analysis
- 1 Hour: Using matplotlib and seaborn on well production data for visualization
- 4 Hours: Using sklearn for regression and classification
- Irreducible saturation prediction from core data
- Rock classification based on well log
- Use of bagging, neighbors, regularization, and support vectors
- 2 Hours: Feature selection, dimensionality reduction, and feature ranking
- 3 Hours: Using sklearn for clustering and outlier detection
- Anomaly detection on porosity-permeability data
- Rock typing (clustering) on well logs
- 1 Hour: Uncertainty quantification for regressors and classifiers
- 2.5 Hours: Advanced regressors and classifiers: neural network and boosting